Unlocking the future of heart transplantation: Non-invasive Diagnostics
Non-invasive Diagnostics will reduce patients’ stress factors and infection risks
Heart transplantation is a highly intricate and critical treatment option for individuals suffering from advanced heart failure who have exhausted all other medical options. If the patient’s heart stops functioning properly and can’t effectively distribute the blood through the body, clinicians might decide to surgically remove and replace it with one from a carefully selected donor.
The field of heart transplantation is continually evolving, with patients in the UK experiencing an average survival rate of 14 years post-procedure, as of 2022.
Successful heart transplantation can greatly enhance a patient's quality of life by allowing them to engage in more physical activity, experience less fatigue, and even start a family. However, it is important to note that the procedure also comes with a range of potential complications, with the rejection of the heart being a common issue.
Rejection occurs when the body recognises the heart allograft as a foreign object and attacks it with an excessive immune reaction.
Currently, this process is monitored through invasive biopsies when a small piece of the transplanted tissue is regularly removed and histologically examined for signs of rejection.
These small surgeries are putting more stress on the patient’s body and can lead to further complications including vein damage or bleeding.
Furthermore, their diagnostics accuracy is disputable. Therefore, recognising the unmet clinical meet, we are developing a non-invasive, accurate and personalised diagnostics tool for heart transplant patients.
Proof of concept Machine Learning with University of The West of England
In a continuation of the KEEP+ programme funded by ERDF (see our earlier blog), the Santorini Scientific team in collaboration with the University of The West of England, recently completed the proof-of-concept stage of Machine Learning-based diagnostics to predict the rejection status of patients.
We have trained our model to analyse patients’ multimodal data while all of it can be measured non- or minimally- invasively, either through collecting standard clinical diagnosis or blood samples.
We used the power of Artificial Intelligence to analyse complex relationships present in the input data and based on that estimate the patient’s current rejection level with an accuracy of over 90%. Multiple techniques are being used to ensure the tool is trustworthy, reliable and suitable for the healthcare setting.
Next phase of the project towards a promising future
Our Machine Learning project is still in its infancy, yet we have an ambitious plan for its future. Our continuous discussions with clinicians and scientists help us stay on track with current medical needs.
Our company is currently preparing to extend the model training database and analyse data from multiple historical sources – medical records of heart transplant patients. Looking back at the patient’s past information will help us to look ahead and see how we can tackle complex data, so our tool can be used in UK hospitals soon.
We are also establishing connections to collect our own data to improve the model’s accuracy and progress towards a promising future.
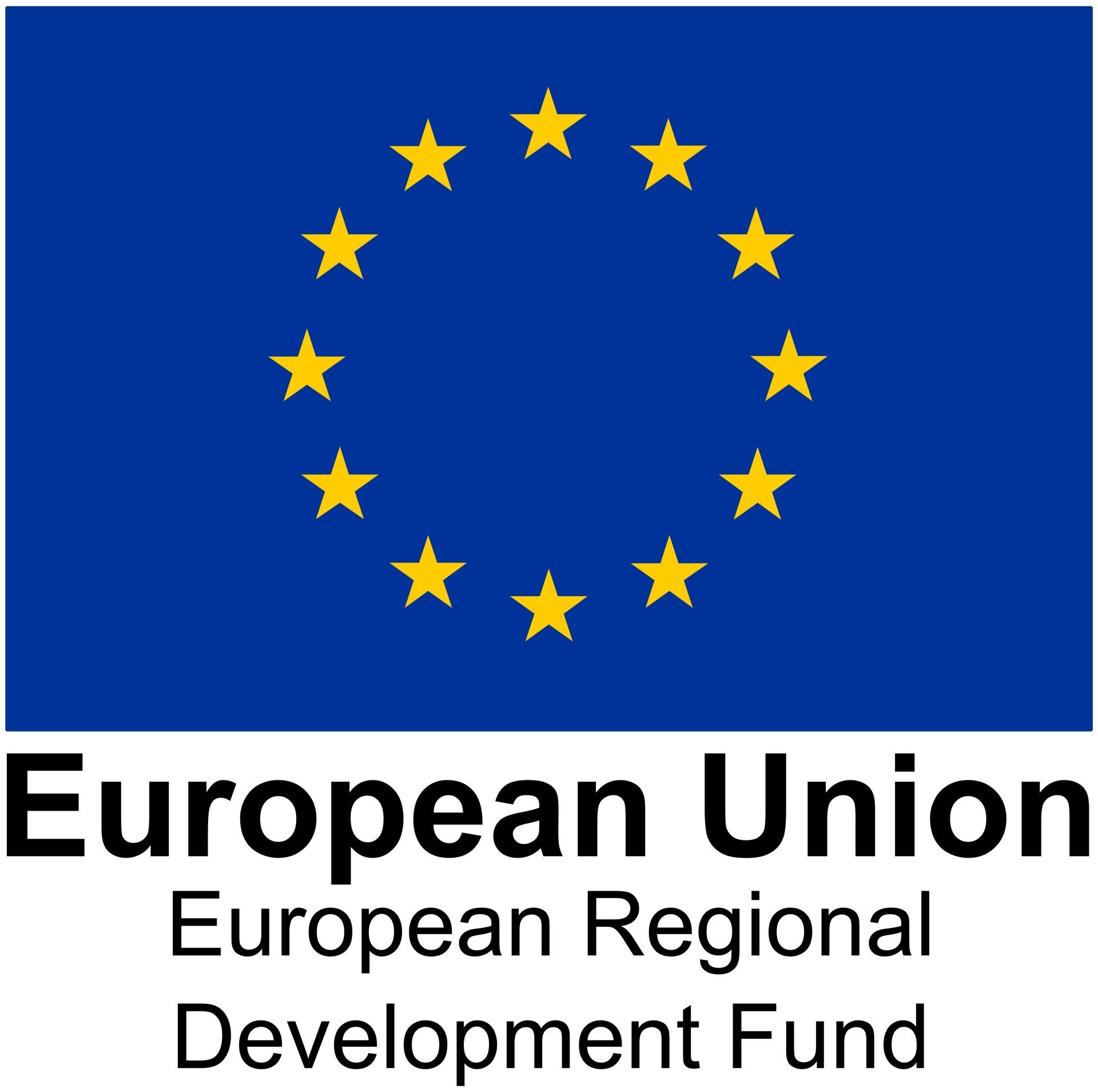
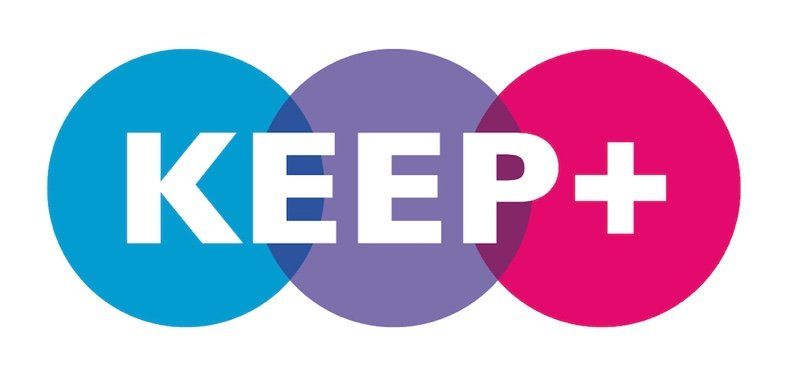